Lending
What's the Secret to Becoming an Exceptional Lender?
Whether it’s loans for individuals or businesses, community banks’ lending activities keep America running. These high-performing community bank lenders, representing a diverse range of communities and specialties, tell us how they use innovation and relationship-based banking to support their customers’ dreams.
Payments & Tech
Instant Payments Insights Every Community Bank Needs

Read the Digital Edition
- Secrets behind successful lending
- Rising from teller to leader
- Rebuilding after California wildfires
Sponsored Articles
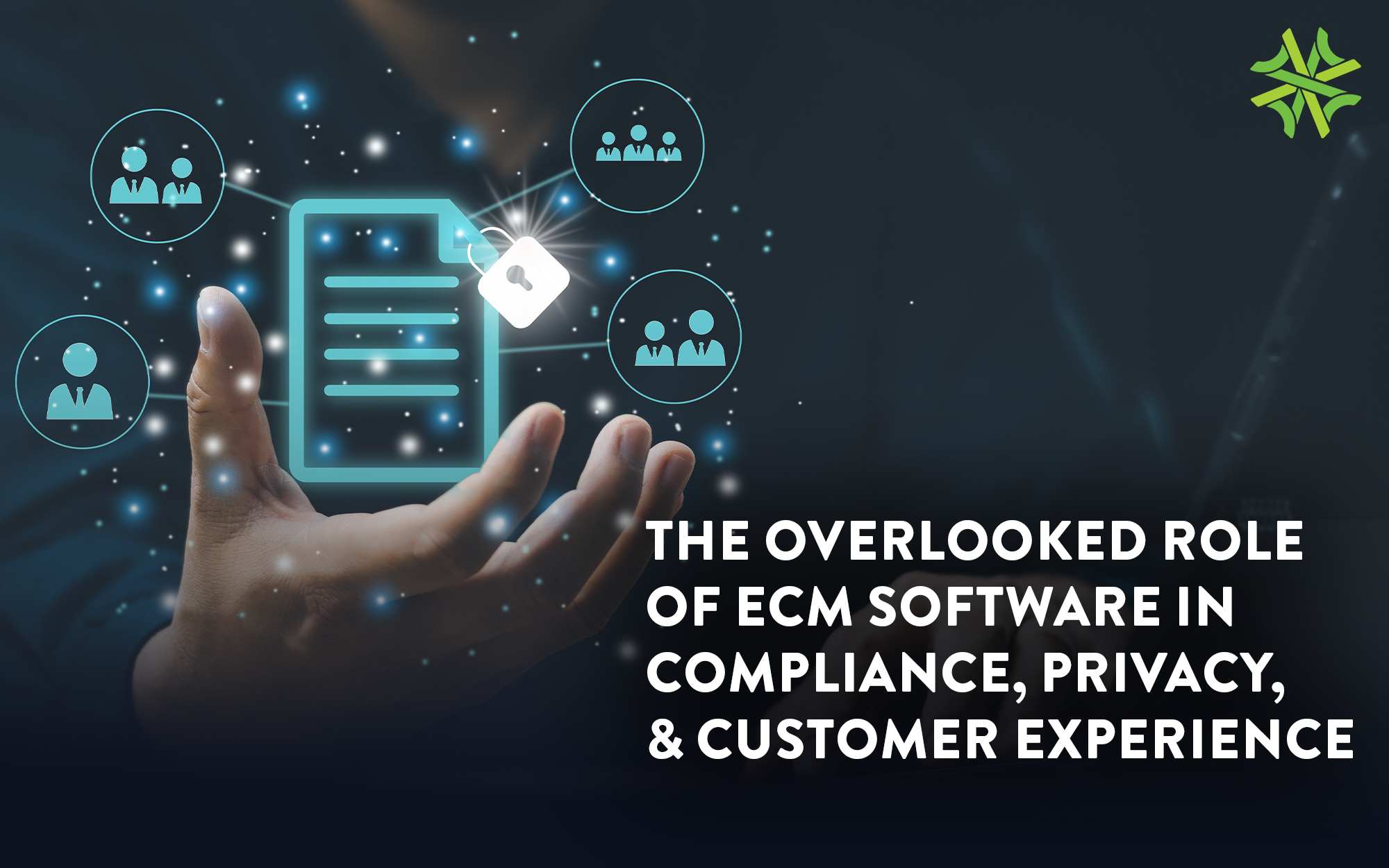
The Overlooked Role of ECM in Compliance, Privacy, and Customer Experience
SponsoredMain Street Matters
Advocacy: Industry Issues
At Halfway Point of 2025, ICBA Marks Significant Advocacy Successes
Financial Analysis
Vigilante justice?
Executive Leadership/C-Suite
Navigating Employee Retention: Staying Competitive in a Changing Market

Be the first to know about the latest issue!
Sign up for Independent Banker eNews to receive twice-monthly emails that alert you when a new issue drops and highlight must-read content you might have missed.
Latest Insights
Compliance & Risk
Creative Solutions for Fortifying ATM Defenses
Payments & Tech
Payments & Tech
Instant Payments Insights Every Community Bank Needs
Payments & Tech
Jacob Eisen: A Can't-Miss Business Card Opportunity
Payments & Tech
Maximizing Profitability with Today's Point-of-Sale Tech
Payments & Tech
Charles Potts: Turning Strategy Into Success With the Right Tech
Columns
Lending
Compliance & Risk
Compliance & Risk
Creative Solutions for Fortifying ATM Defenses
Compliance & Risk
The Overlooked Role of ECM in Compliance, Privacy, and Customer Experience
Sponsored
Compliance & Risk